Technology
We design and create microbial production strains with ArtemisAI, our predictive platform combining artificial metabolic networks (AMNs), machine learning, and constraint-based modeling. By simulating strain performance in silico, we cut development time and boost success at scale—making biology a reliable design tool for fermentation-based products.W
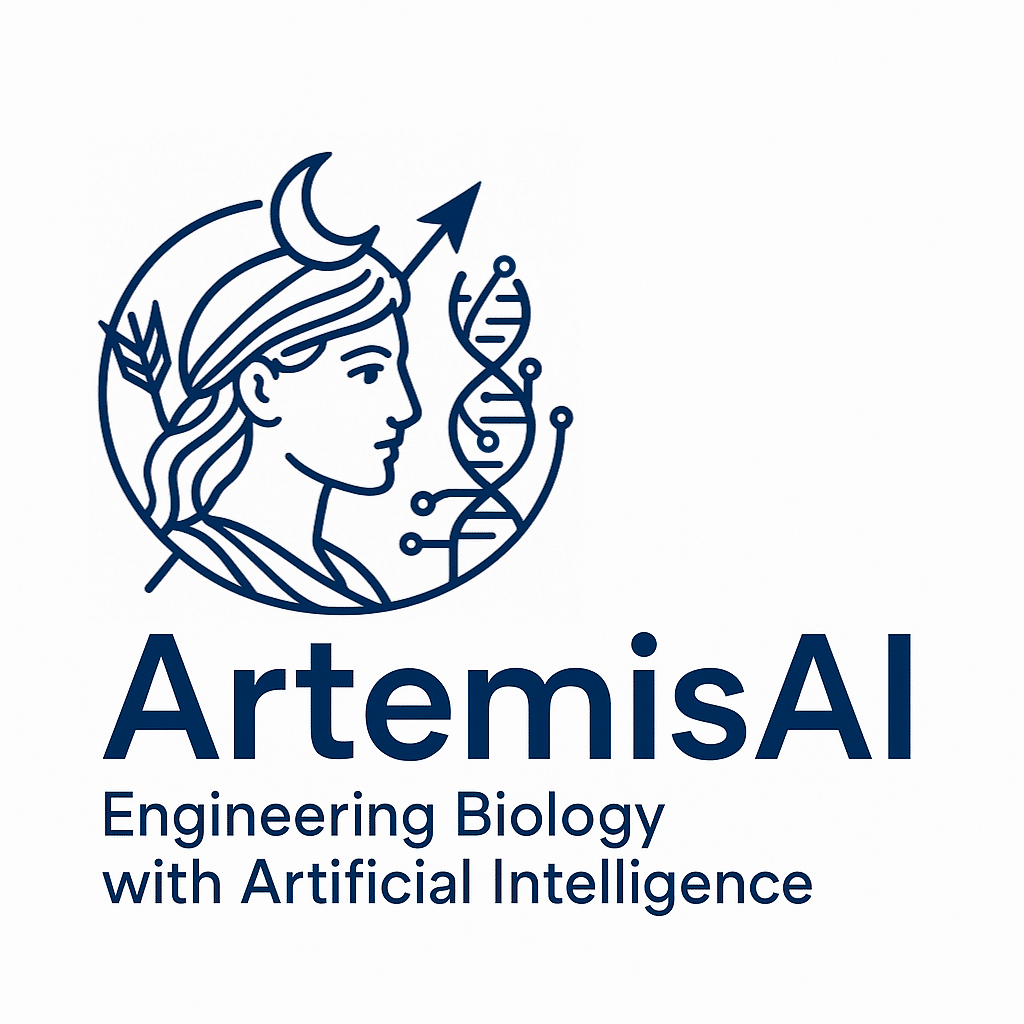
Artemis AI
Most biotech tools rely either on constraint-based modeling, which is biologically grounded but limited in predictive scope, or on machine learning, which can uncover patterns in large datasets but lacks interpretability and often needs huge volumes of training data. ArtemisAI brings the best of both worlds together.
By combining artificial metabolic networks (AMNs), machine learning, and mechanistic modeling, ArtemisAI builds digital twins of microbial cells and fermentation processes that are both explainable and predictive. This means we can simulate and optimize microbial performance before entering the lab, designing strains that work not just in theory—but in real-world, industrial conditions.
The result: faster development cycles, and higher success rates when scaling. Whether we’re producing nutraceuticals, specialty ingredients, or pharmaceutical intermediates, ArtemisAI gives us—and our partners—a decisive edge in turning microbes into efficient, scalable production platforms.
From Idea to Market
In silico strain prototyping drastically reduces the number of wet lab iterations needed.
Predictive simulations identify scalable designs early, avoiding costly false positives.
Faster go/no-go decisions based on biologically interpretable outputs and metabolic bottlenecks.
Seamless integration with fermentation parameters allows process-aware strain development.
Shorter development timelines enable quicker product validation and earlier revenue.
Optimizing Margins at Industrial Scale
Yield and productivity improvements via pinpointed pathway redesigns.
Custom media optimization guided by simulated metabolic demands, lowering input costs.
Process tuning support by predicting strain performance under industrial fermentation conditions.
Reduced batch failures and variability through robust, well-characterized designs.
Continuous improvement cycles enabled by digital twin modeling of fermentation outcomes
With ArtemisAI, biology becomes a strategic asset—designed for performance, built for scale, and optimized for profit.
ArtemisAI directly drives ROI by reducing development costs, shortening time to market, and increasing the likelihood of success at scale. With fewer failed iterations and faster validation, partners gain faster access to revenue-generating products. It also enables new business models—like milestone-based royalties and joint ventures—by delivering high-performing, scalable strains that de-risk commercialization from the start.
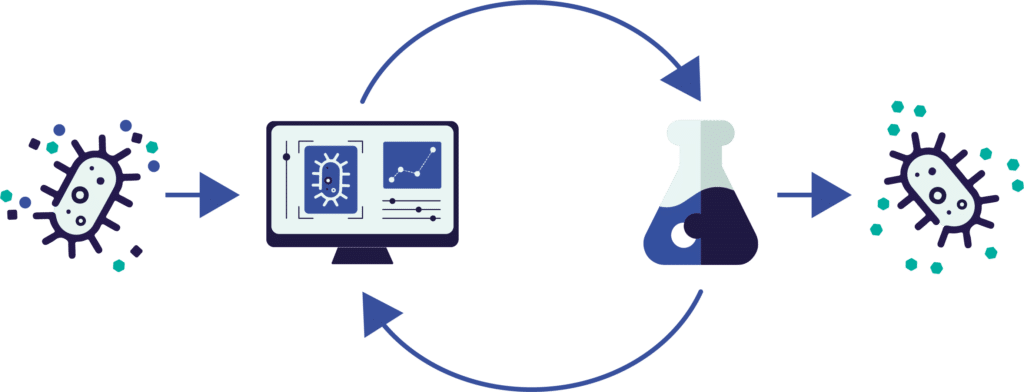
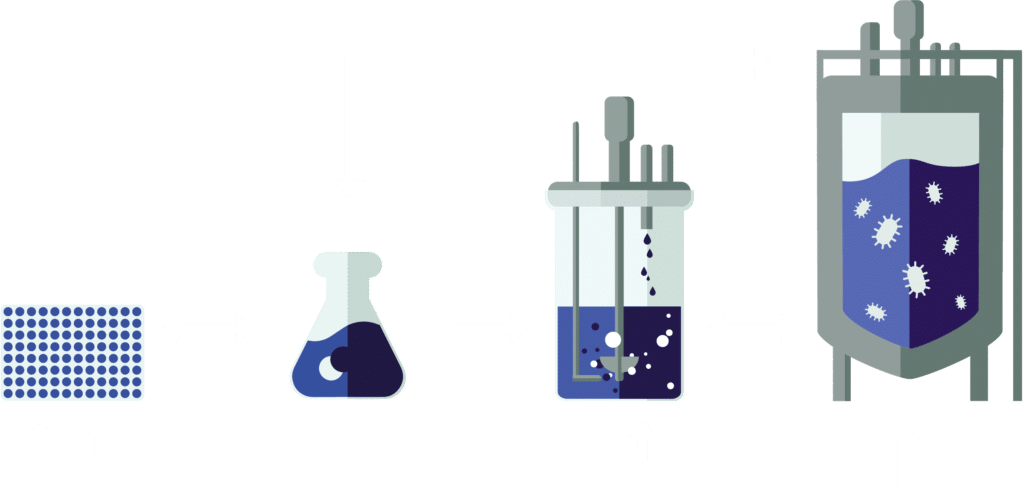
Modelling over different scales greatly speeds up strain development
ArtemisAI is built to guide success from microtiter plate to industrial fermenter. It models microbial behavior across scales, allowing us to predict how a strain will perform not just in controlled lab conditions, but in real-world bioreactors. From selecting the right pathways at microliter scale to optimizing productivity and media at pilot and manufacturing volumes, ArtemisAI reduces scale-up surprises and ensures that what works in the lab is ready to perform at industrial throughput.
Design smarter: Predict the best gene combinations before you build.
ArtemisAI accelerates genetic design by simulating and scoring thousands of gene and pathway combinations before they enter the lab. Using artificial metabolic networks, it predicts how different genetic edits will affect growth, yield, and productivity—allowing us to test design performance virtually and select only the most promising constructs for experimental validation. This dramatically reduces the number of builds needed, saving time and resources while increasing the chance of success.
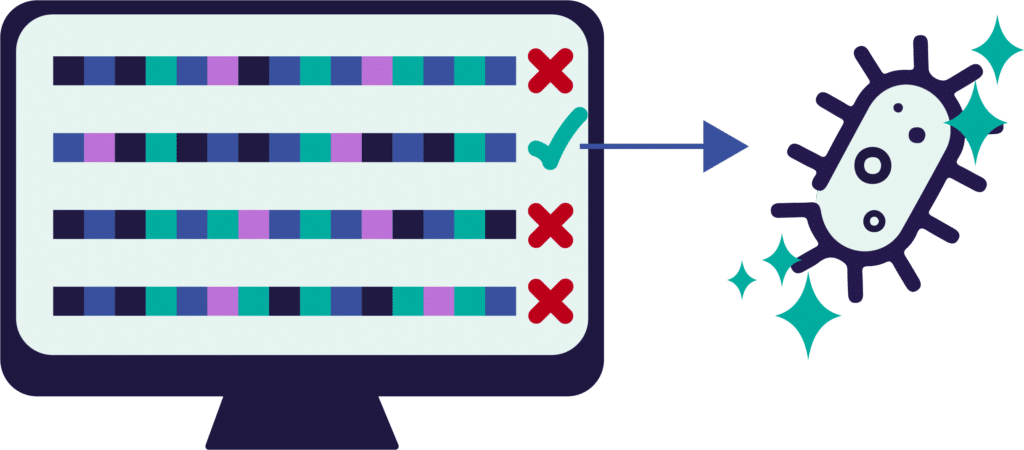
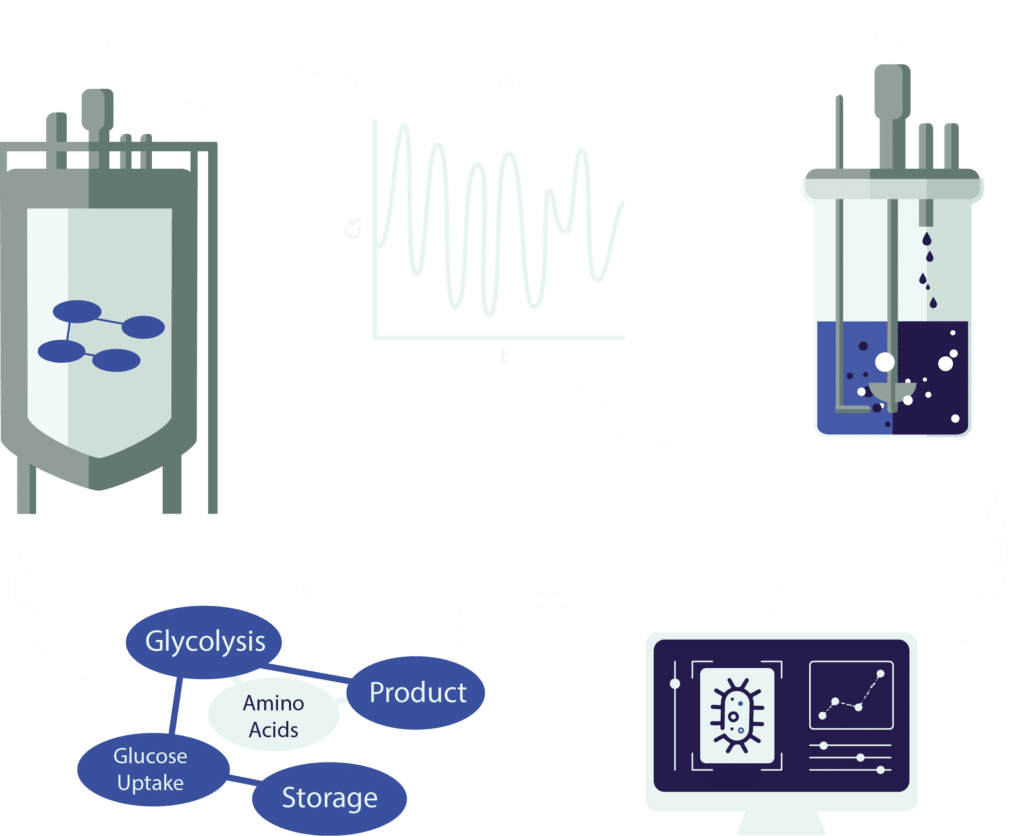
Scale down fermentation simulation
Comprehensive data collection in large-scale reactors is extremely challenging. Computational fluid dynamic models, however, simulate heterogeneous conditions, aiding experimental design. Simulations can be used to replicate glucose concentration gradients in bench-top reactors to study transcriptomic responses.
Figure
A: A coupled spatial model of a reactor with a pooled metabolic model is used to simulate the B: conditions a cell encounters throughout the reactor. C: These conditions are replicated in bench-top reactors to collect D: data on large scale conditions. E: After data analysis, the metabolic model can be improved and used in a new experimental cycle.
Example: Strain engineering to enable low-cost carbon alternatives
While fermentation can be a green alternative to petroleum-based production, the need for high-quality carbon sources can contribute significantly to cost and increase demand on food-based crops. Strain engineering can enable the use of low-cost carbon sources derived from waste products. For example, the expression of amylase enzymes in yeast can allow for growth on starch derived from plant waste. Alternatively, with our media optimisation tool we can predict how to utilise a side stream as carbon source for the microorganism.
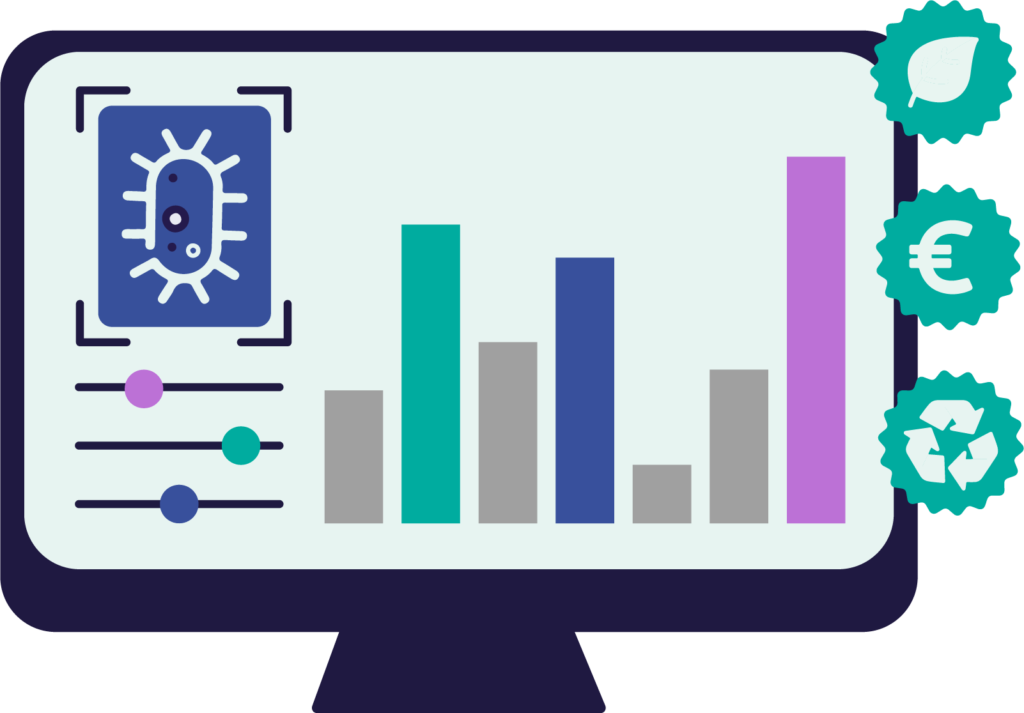